Disease trajectories following myocardial infarction
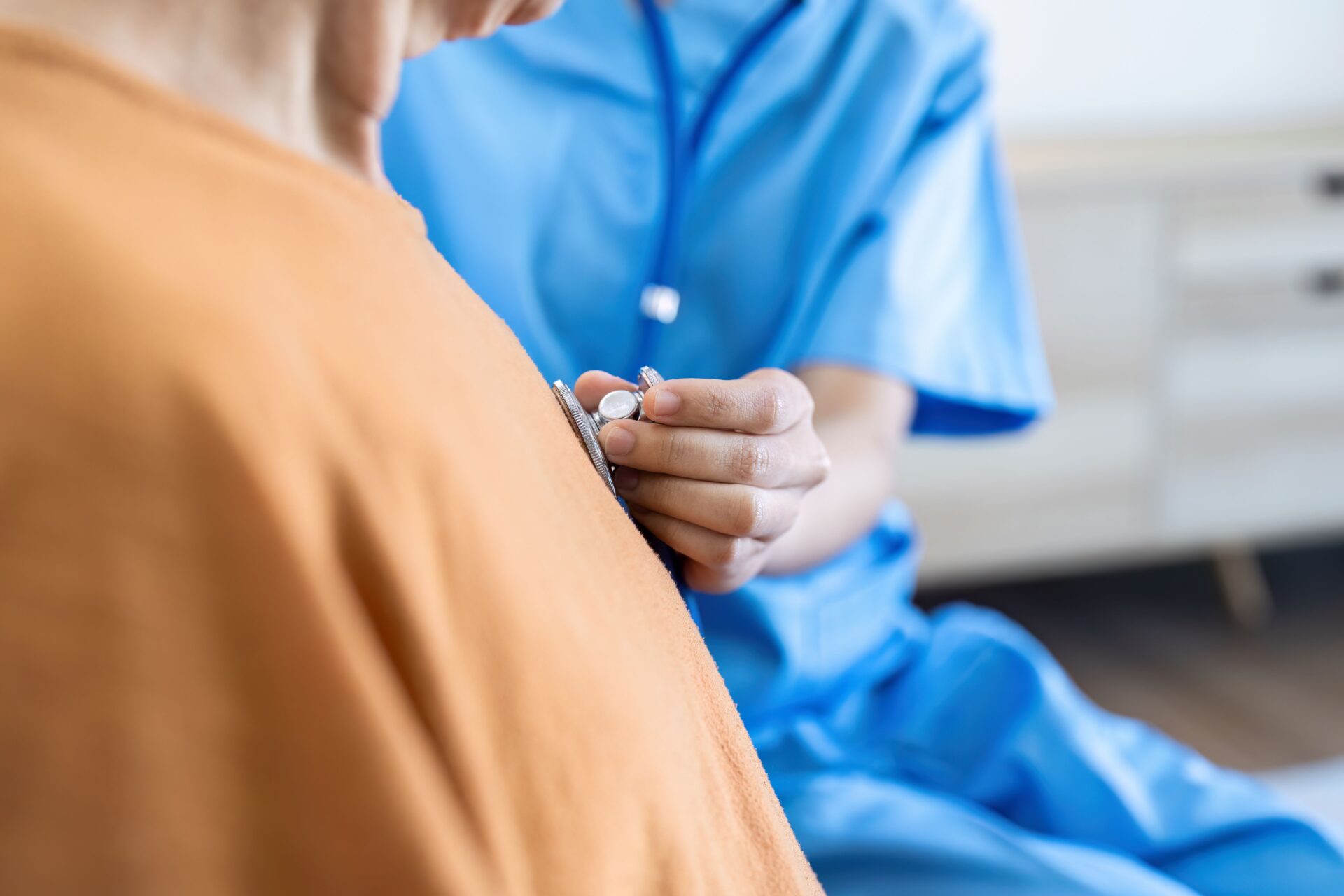
Background
Major advances in our understanding and treatment of disease mean that it is now common to survive over a decade after the diagnosis of myocardial infarction (heart attack). However, this shift change in survival means there are an increasing number of individuals vulnerable to the development of adverse health outcomes as they live longer. This can include frequent readmission to hospital, the accrual of new disease states, reduction in quality of life, increased healthcare-associated costs, greater dependency, and premature mortality.
The full extent of which conditions are likely to develop following a heart attack is unknown —yet the number of survivors of MI has increased dramatically in recent decades.
One of the major barriers to our understanding of sequences of disease accrual is a lack of appropriate methods. Current statistical approaches which model multiple diseases rely on existing knowledge of the diseases following a heart attack, the order in which we expect conditions to occur and do not work well for many diseases at once. This therefore limits scientific discovery of previously unknown consequences of a heart attack.
Dr Marlous Hall and her team investigated temporally ordered sequences of thousands of unique disease codes at unstructured time intervals following MI in nationwide electronic health record data. The team of epidemiologists, bioinformaticians, cardiologists and data scientists worked together to discover patient-level disease trajectories following MI in a nationwide cohort study of 145 million hospitalisation episodes for 34 million individuals across England through the novel application of process mining.
In doing so, they have established a taxonomy of post-MI temporal disease profiles, simultaneously capturing the combination of disease states, their temporal sequence, their relative risk, and association with long-term survival compared with non-MI individuals. These results provide healthcare professionals—as well as patients and their carers—with detailed insight into the likely course of disease following MI. This study can be used to further highlight the most pertinent early intervention points to interrupt disease pathways, reducing premature morbidity and mortality among the growing cohort of survivors of MI.
The study was funded by a BHF-Turing Data Science Award which promotes multi-disciplinary research to generate data science solutions to key cardiovascular problems.
Methods & findings
This was a national retrospective cohort study of all hospitalisations (145,670,448 episodes; 34,083,204 individuals) admitted to NHS hospitals in England (1st January 2008–31st January 2017, final follow-up 27th March 2017) using Hospital Episode Statistics (HES) data linked with mortality records from the Office for National Statistics (ONS).
Data were stored and analyses conducted within the Leeds Analytics Secure Environment for Research (LASER) – a bespoke trusted research environment developed by the Leeds Institute for Data Analytics. Importantly – this platform provided the necessary high level of security requirements for these sensitive NHS Data as well as providing the computational power required for analyses of this complexity and size.
Through process mining, 28,799 unique disease trajectories of all major disease diagnoses following MI were identified. In order to understand the course of disease following MI over and above the effects of ageing and disease development during the normal life course - all post MI trajectories were compared with a risk-set matched control cohort of individuals of the same age and sex hospitalised in the same NHS trust at the same time as individuals with MI.
The accrual of multiple circulatory diagnoses was more common amongst MI patients (RR 4.32, 95% CI 3.96–4.72) and conferred an increased risk of death (HR 1.32, 1.13–1.53) compared with matched controls. Trajectories featuring neuro-psychiatric diagnoses (including anxiety and depression) following circulatory disorders were markedly more common and had increased mortality post MI (HR ranging from 1.11 to 1.73) compared with non-MI individuals.
The full study methods and results are available via our open access publication in EBioMedicine and results as well as programming code can be explored interactively via our bespoke web-application designed in support of the research via GitHub.
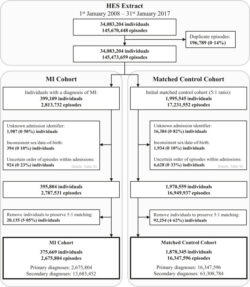
Fig. 1 Derivation of myocardial infarction (MI) and risk-set matched control cohorts from Hospital Episode Statistics (HES) hospitalisation episodes for adults over the age of 18 in England, 2008–2017.
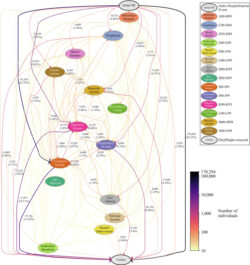
The network of disease transitions for temporally sequenced diseases following myocardial infarction (MI), for adults with MI in England, 2008–2017. Directed edges between chapter headings represent progression of individuals from one primary diagnosis to the next, grouped by ICD-10 chapters. Disease trajectories from all individuals within the MI cohort (n = 375,669) are used to construct this network. The number of individuals (and percentage of cohort) who progress from one disease chapter to the next is shown when at least 1% of individuals contribute to that progression. Edge colour (ref: colour-bar) and thickness are proportional to the number of individuals that progress from one chapter to another. Index Hospitalisation Event is defined as the first primary diagnoses (MI) at the time of matching. An interactive version of this figure—showing counts for all connections; the network for the secondary analyses; and granular sub-chapter transitions—is available at https://multimorbidity-research-leeds.github.io/.
Interpretation
These results provide an opportunity for early intervention targets for survivors of MI—such as increased focus on the psychological and behavioural pathways—to mitigate ongoing adverse disease trajectories, multimorbidity, and premature mortality.
Funding
British Heart Foundation; Alan Turing Institute.