Extracting actionable insights from free text police data
Alex Coleman, Daniel Birks, Nick Malleson, Graham Farrell - University of Leeds
Can creative writing catch criminals?
When a crime occurs large amounts of information are captured within the narrative description of the incident. This data contains useful information that is not fully utilised at present due to its unstructured nature.
Project aims
Using text mining and natural language processing methods to determine whether actionable insights could be derived from crime narrative data. The project asks, is it possible to identify crime types by the report narratives? Do these narratives provide information regarding the modus operandi (MO) of the offender? Can emerging crime MO be identified from crime narratives?
Explaining the science
The approach used a topic modelling algorithm, Latent Dirichlet Allocation (LDA). This approach identifies latent topics within documents by determining a probability distribution of words likely to occur together within a latent topic. We performed LDA on a processed corpus of documents provided by Safer Leeds and then labelled documents by their most dominant LDA topic.
Results
We developed a robust, reproducible methodology for using LDA topic modelling to identify specific MOs from police free text data. This approach was exploratory and using the data provided by Safer Leeds was able to identify 21 MOs from within Burglary Dwelling data. Reports were clustered into these 21 MOs and used as a data source for a Shiny application (image shown) that Safer Leeds can use to observe in space and time thematic trends in crime behaviours to help aid crime prevention.
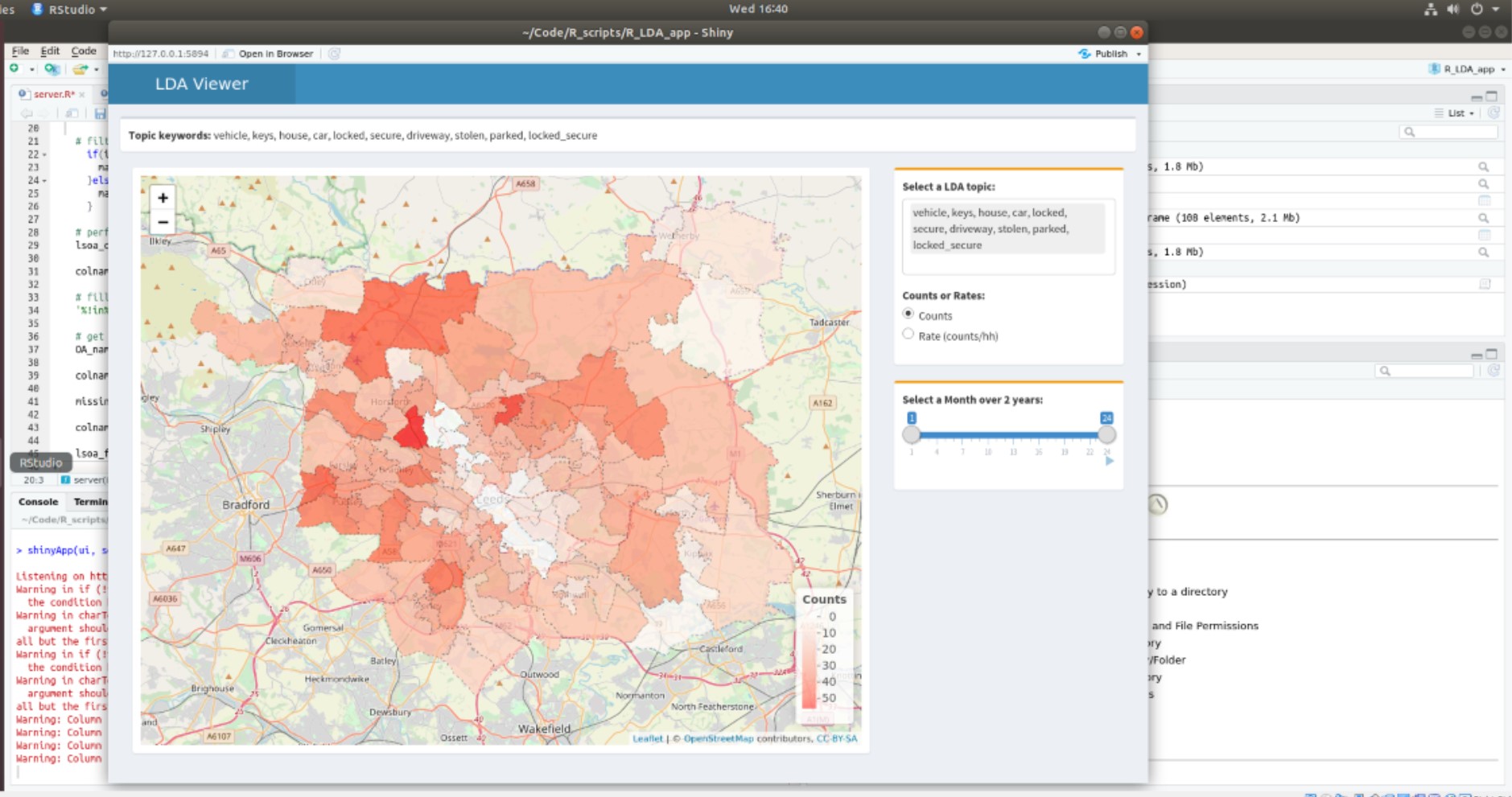
A screenshot of the app visualising the spatial-temporal clustering of a topic generated by topic modelling.
Applications
This approach could be refined and implemented as an automated approach to determining more specific crime categories or implemented in real-time to identify emerging crime MOs.
“Vast amounts of rich unstructured text data are collected by police and their partners on a day-to-day basis. These large datasets present significant analytical challenges, but also offer huge opportunities. The work we’re doing with LIDA will help us harness this resource to better understand and ultimately, we hope, reduce crime.” // David Jackson, Partnership Intelligence Lead, Safer Leeds
Funders / Partners
This project was supported by David Jackson at Safer Leeds who provided the text data and gave input on the project, and was also supported by Wave 1 of The UKRI Strategic Priorities Fund under the EPSRC Grant EP/T001569/1, particularly the “Criminal Justice System” theme within that grant & The Alan Turing Institute.