Forecasting the Future: Pioneering Weather Predictions with Machine Learning
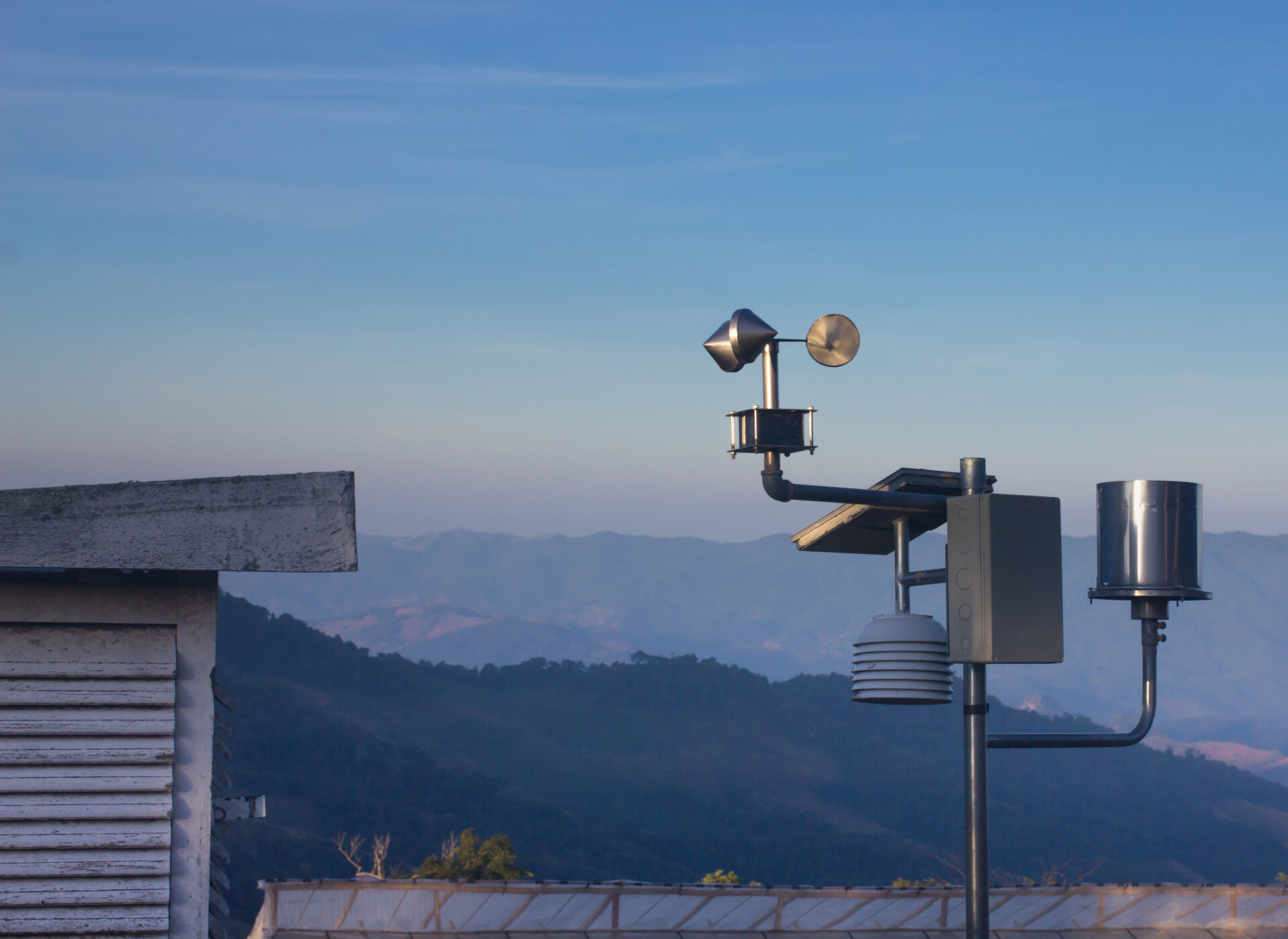
by Maria Luisa Taccari
On Monday, 30 Oct 2023, The Alan Turing Institute (the UK's national institute for data science and artificial intelligence) and the Met Office (the United Kingdom's national weather service) announced a partnership aimed at revolutionizing weather forecasting using artificial intelligence (AI). This collaboration aims to develop AI models that will enhance forecasting accuracy, particularly for extreme weather events, thereby potentially saving lives and protecting infrastructure. In the project's initial phase, researchers will develop a new AI model based on graph neural networks. Subsequently, this AI model will be integrated into the Met Office's existing supercomputing framework to enable frequent comparisons of its effectiveness against the conventional physics-based methods of forecasting that are currently in use.
The shift to data-driven
Traditional numerical methods such as “numerical weather prediction” (NWP), although advanced, have limitations, in particular their accuracy comes at a cost of computational demands. In this context, the last few years have witnessed a shift toward the adoption of data-driven approaches, particularly artificial intelligence, in the realm of weather forecasting. AI integration promises faster models, with training being resource-intensive but only done once. Once trained, these models can analyse new data in real or near-real-time, enabling almost instantaneous predictions. They also have the potential to become sharper and more refined, improving forecast accuracy by identifying complex patterns not easily captured by explicit equations.
This shift was explained by Dr. Mariana Clare from the European Centre for Medium-Range Weather Forecasts (ECMWF) during a talk to our SciML group in Leeds—a collective of PhD students using ML for environmental applications. Dr. Clare highlighted the intrinsic challenges of weather prediction: chaotic dynamics, interconnected earth systems, and our still-developing understanding of many processes.
Nevertheless, the wealth of earth system data provides ample training opportunities for AI models. Dr. Clare described the progress in the field, including GraphCast, a model by Google DeepMind that uses graph neural networks for quick medium-range forecasts, making predictions in under 60 seconds for a 10-day forecast. The speed and the novel use of internal multi-mesh representations for simulating interactions within the atmosphere could be a game-changer, especially considering it outperformed the top NWP-based system in 90% of the variables tested.
The potential of AI
This is the exciting potential of AI—it promises to accelerate our models without significantly compromising on detail. AI's potential extends far beyond this single application, offering abundant opportunities across a wide range of scientific disciplines where AI models could deliver predictions much faster than traditional numerical methods. In my own research, I am developing a similar type of model emulator for a different application: I am creating groundwater emulators in collaboration with the Dutch research institute Deltares.
This work has significant implications for predicting floods and droughts, enabling scenario calculations that would otherwise be prohibitively time-consuming. Similar to weather forecasting, where simulating a model for accurate predictions could take longer than the forecast period itself, the model I am developing could be utilized in daily practices. For example, it could estimate the impact on the local groundwater table of a construction pit before construction begins, a task often not feasible with current numerical models, which can take weeks to process.
AI also shows promise in data assimilation; for example, it could potentially reveal complex patterns from the hundreds of millions of daily observations gathered for weather prediction. Currently, GraphCast and similar models are trained on ERA5 data, which are climate reanalyses combining past observations with models to generate consistent time series on climate variables, but the future could see AI trained on actual raw data. As explained by Dr. Keisler in his recent talk to our SciML group, this is an anticipated next step, potentially offering higher accuracy than current NWP models. The large amount of data in the weather world provides the perfect opportunity to check if numerical models based on physics equations are needed when a large amount of data is constantly available.
However, the integration of AI into forecasting is not without challenges. There is a risk of AI producing highly confident but physically implausible forecasts, and questions remain about its ability to uncover new patterns or if it can generalize to unseen scenarios, such as unfamiliar climate conditions. As the partnership between The Alan Turing Institute and the Met Office progresses, it's vital to balance the capabilities of AI with a solid understanding of the science behind it.
Redefining our relationship with weather prediction
Looking ahead, it's intriguing to think about the potential impact of faster AI models on our understanding of weather physics and decision-making processes. The key advantage of these models is that once trained offline, they don't require massive computing power during the online phase, enabling them to run on relatively modest hardware, such as desktop computers. This accessibility suggests that in the near future, accurate weather models and simulations could be used not only by meteorologists but also by a wide range of individuals, companies, and other entities.
The ability to run these simulations quickly and easily could dramatically change how we respond to weather events, enhancing everything from daily planning to emergency response strategies.
It would be intriguing to see the partnership between The Alan Turing Institute and the Met Office explore not only the development of better and faster models but also the broader implications of these advancements. Questions about what kinds of decision-making processes AI-enabled weather forecasting could enhance, and where traditional numerical weather forecasting might still be preferable, are equally important. I hope that this partnership goes beyond merely creating new models; it's about fundamentally redefining our relationship with weather prediction. Understanding how AI can optimally benefit humanity, particularly in an era marked by rapidly changing and uncertain climatic conditions, is a central theme of this collaboration.
Maria Luisa Taccari is currently pursuing her PhD at the University of Leeds, focusing on the development of deep learning surrogate models in groundwater flow simulation. Maria Luisa holds a BSc from the University of Padova and an MSc from TU Delft University, both in Civil Engineering and awarded cum laude. Prior to her PhD, Maria Luisa had five years of industry experience working at the Dutch research institute, Deltares.