Artificial intelligence in ovarian cancer histopathology: a systematic review
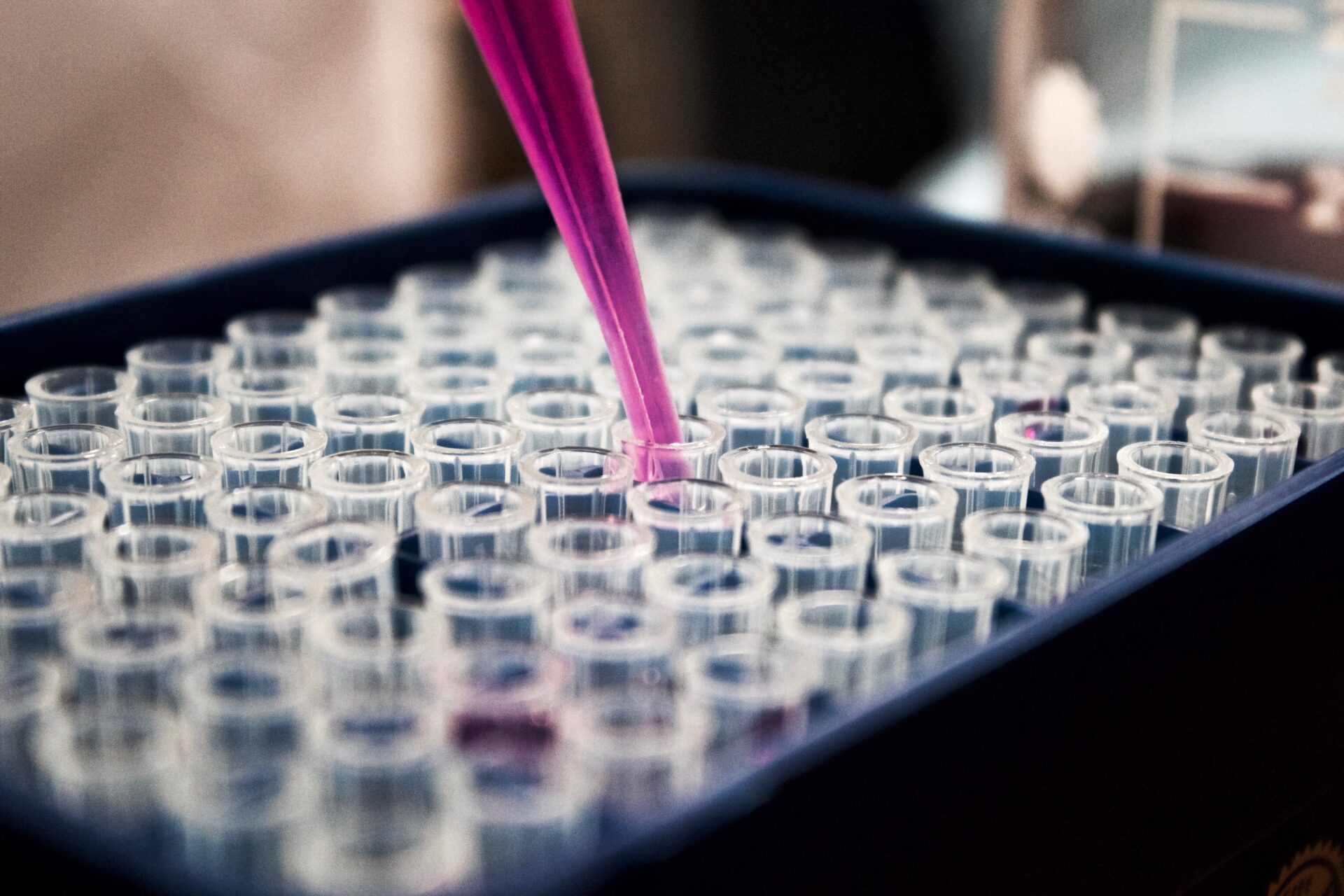
Ovarian cancer is the eighth most common malignancy in women worldwide. It is notoriously difficult to detect and diagnose, with ineffective screening and non-specific symptoms similar to those caused by menopause. Encompassing primary malignant tumours of the ovaries, fallopian tubes, and peritoneum, the disease has often started to spread within the abdomen at the time of diagnosis (FIGO4 Stage 3). This typical late stage at diagnosis makes ovarian cancer a particularly deadly disease, with the 314,000 new cases diagnosed each year translating to 207,000 deaths per year globally.
This research is about using artificial intelligence (AI) to help diagnose and predict outcomes in ovarian cancer by analysing tissue samples. The research looked at a variety of studies found in medical databases PubMed, Scopus, Web of Science, Cochrane CENTRAL, and WHO-ICTRP up to May 19, 2023, and focused on studies that used AI to make predictions about ovarian cancer in human tissue images. The study was registered on PROSPERO (CRD42022334730) and PRISMA 2020 reporting guidelines were followed.
Fig. 1: PRISMA 2020 flowchart.
PRISMA 2020 flowchart of the study identification and selection process for the systematic review. Records were screened on titles and abstracts alone, and reports were assessed based on the full-text content. CENTRAL Central Register of Controlled Trials. WHO-ICTRP World Health Organisation International Clinical Trial Registry Platform.
The research found 1,573 studies, but only 45 of which met the criteria. These 45 studies involved 80 different AI models. Some of these models were for diagnosing ovarian cancer, some for predicting how it might progress, and others were for various related purposes. The AI models used different amounts of tissue samples from 1 to 1,375 slides and 1 to 776 patients with ovarian cancer.
However, the research discovered issues with the quality of these studies with significant bias related to a lack of thorough analysis and incomplete reporting, particularly concerning how the participants for the studies were selected or recruited. The studies did not provide enough information or transparency about how they chose the people who participated in the research, which could potentially affect the reliability and accuracy of the study results.
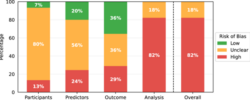
Fig. 2: PROBAST risk of bias results.
PROBAST risk of bias results summarised for the 45 papers included in this review.
Fig. 2: PROBAST risk of bias results.
PROBAST risk of bias results summarised for the 45 papers included in this review.
To make AI more useful in diagnosing and predicting ovarian cancer, the researchers recommend that future studies should be more transparent about how they got their data and how they built their AI models. They also suggest better ways to test the models to make sure they work well. This research was supported by the Engineering and Physical Sciences Research Council.